How do you establish product metrics to evaluate success?
Short answer: Identify your high-level engagement metric, form hypotheses about how you will improve it, then identify a proxy metric for each of these hypotheses (e.g. your product strategies).
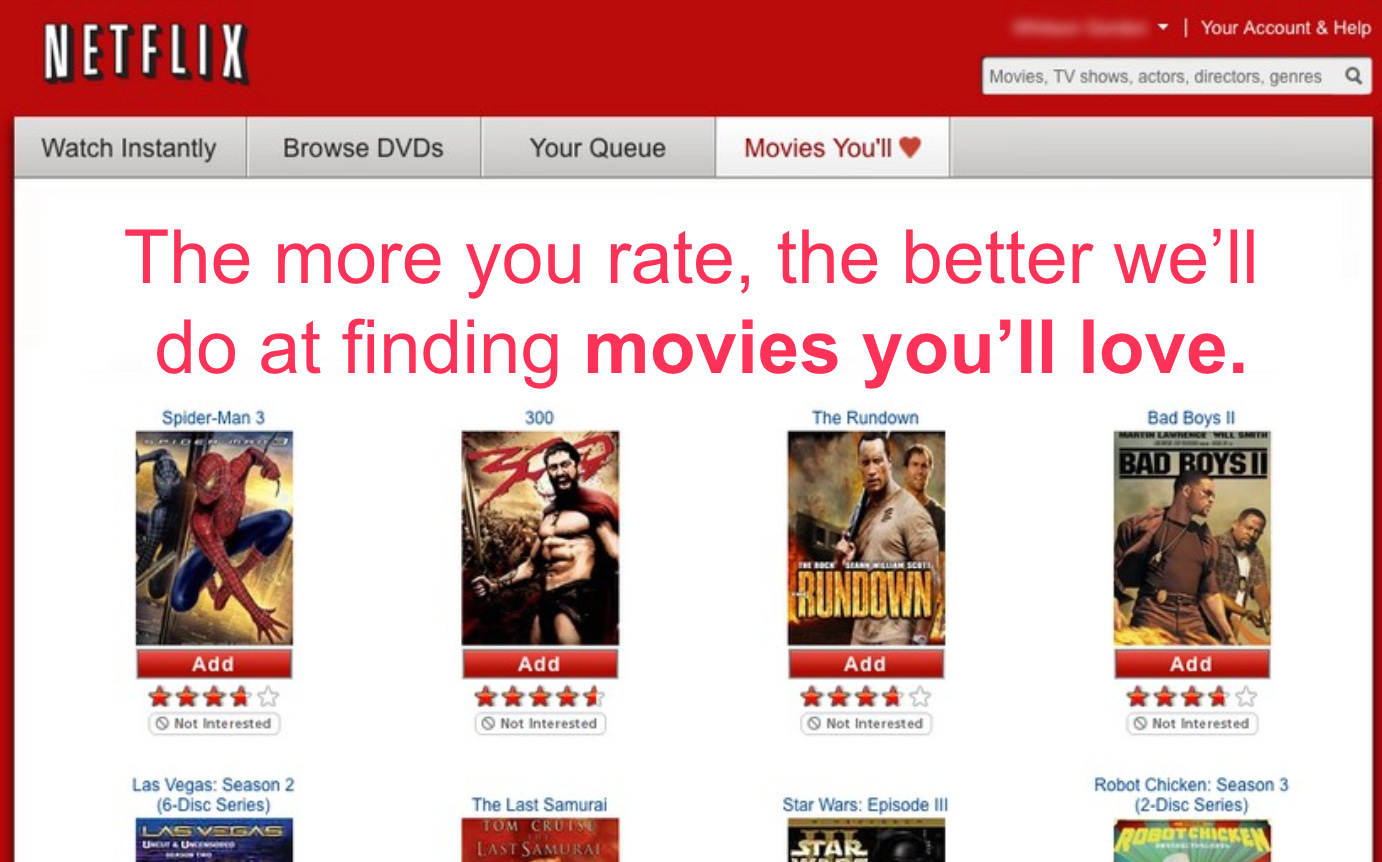
Here are my upcoming public events and special offers:
Click here to purchase my self-paced Product Strategy course on Teachable for $200 off the normal $699 price — the coupon code is 200DISCOUNTGIBFRIENDS but the link will handle this automatically. (You can try the first two modules for free.)
New podcast episode: “One Knight in Product” with Jason Knight
New! I’m on Instagram at “AskGib”
4/29 “Inventing the Future (Is Hard)” with Crisp (5 PM CET/9 AM PT)
5/27 “Hacking Your Product Leader Career” Masterclass with Productized (6:30 am PT, 3:30 pm CET)
Welcome to the 250 new members who joined this past week! After less than four months, we’re 4,300 strong. Each week, I answer a few “Ask Gib” questions, drawing from my experience as VP of Product at Netflix and Chief Product Officer at Chegg (the textbook rental and homework help company that went public in 2014). Today’s answer is essay #47!
To become part of the “Ask Gib” community, subscribe now — this way, you’ll never miss an essay:
How do you establish product metrics to evaluate success?
When I meet a new company, I ask the exec team to force-rank growth, engagement, and monetization. I also ask them to identify a metric for each of these three factors. There’s a lot of focus on the engagement metric— the high-level metric that measures product quality. Then we outline various product strategies to move our engagement metric. Last, we develop a proxy metric for each of these hypotheses.
Below, I illustrate this process for Netflix then conclude with a detailed example describing the proxy metric for their personalization strategy.
1) The GEM model
As I work with a company, I ask the executive team to force-rank three factors: Growth, Engagement, and Monetization. (GEM refers to the first letter of each word.)
Growth measures the annual growth rate. This year, Netflix will have 20% year-over-year member growth.
Engagement measures product quality. Monthly retention is Netflix’s high-level engagement metric. Today, only 2% of members cancel each month. (In 2002 it was 10%.)
Monetization measures economic efficiency. Netflix measures the lifetime value of a customer (LTV), which is close to $300.
I ask the team to force-rank these three metrics. There’s no correct answer, and the exercise inspires debate. The important thing is everyone agrees on the forced-rank priorities of “GEM” across the company. (Today, I’d guess that Netflix prioritizes the three factors: 1. Growth 2. Monetization 3. Engagement.)
I begin with the “GEM” exercise for three reasons:
To translate efforts into metrics we can measure.
To open up the conversation about how the team measures high-level product quality — the product team’s engagement metric.
To build alignment. Force-ranking the three factors helps the organization to align against a prioritized set of high-level metrics.
For a product organization, the most important metric is the high-level engagement metric.
Your high-level engagement metric
Ask yourself, “What’s the one metric I use to assess product quality?” Most companies fit into one of three models:
Ad-based companies use monthly active users (MAU) in the early days, then shift to daily active users (DAU) as the product achieves broader reach.
Subscription-based companies seek customers who pay for the service on a monthly/annual basis. Retention is an excellent proxy for product quality at these companies.
eCommerce companies focus on transactions. Their engagement metric focuses on a set of customers who make a minimum number/dollar value of paid transactions each month, quarter, or year.
What about the concept of a “North Star” metric? I choose not to use this phrase as it creates confusion. It implies that there is one metric that drives everyone in the company or product team, and it’s rarely that simple.
The closest thing to a North Star metric for me is the high-level engagement metric. But in many cases, an exec team prioritizes engagement second or third, which creates confusion because everyone expects the North Star metric to be the highest priority. Instead, I use the phrase “high-level engagement metric” to describe the metric that measures product quality.
2) How to identify your proxy metrics
A high-level engagement metric's challenge is it’s both hard to move and consequently glacially slow to change. Netflix retention, for instance, improved from a 5% monthly cancel rate in 2005 to a 2% cancel rate today-- that’s only one percentage point improvement every five years.
It helps to have more sensitive metrics to measure your progress and validate your hypotheses about how you hope to improve retention. Proxy metrics are a more sensitive stand-in for your high-level engagement metric. Think of them as leading indicators for hoped-for progress against your high-level engagement metric.
What are your hypotheses to improve retention?
Imagine you’re the product leader at Netflix. What are your theories and hypotheses about how you will improve retention? Here’s a list of hypotheses from 2005:
A more personalized experience
A simpler experience
Faster DVD delivery
Faster movie delivery via streaming
Exclusive content
Movie ideas from friends
A more entertaining experience
Unique movie-finding tools
Proxy metrics exist to help determine whether your hypotheses will improve the high-level engagement metric. In the long-term, you hope to move your proxy metrics “up and to the right” and demonstrate that these improvements lead to progress against your high-level engagement metric. Eventually, you want to prove that the proxy and engagement metric relationship is both correlated and causal.
3) An example: Netflix’s personalization metric
What’s the proxy metric for the hypothesis that a more personalized experience will improve retention? I’ll begin with some general criterion for proxy metrics:
The data exists and is available to you.
The proxy is sensitive enough that you can affect it in the short term.
It measures consumer and, potentially, shareholder value. (There’s an opportunity for margin enhancement.)
In the context of an A/B test, it describes which experience is “better.” The proxy helps to measure changes in customer behavior as observed in AB tests.
It’s not an average. The danger of averages is that you can change the experience of a small subset of customers a lot — driving up the average— but not improve the overall experience for the vast majority of customers. You’re generally better off structuring the metric as a threshold — % of customers who do at least (X) of (new feature).
It’s not gameable. For example, the initial proxy metric for customer service at Netflix, “Customer Contacts per 1,000”, was easy to game— you could hide the 800 number on the site. So we amended the metric: “Contacts per 1,000 with the 800 number available within two clicks.”
It’s independent. Efforts against the specific hypothesis impact the proxy metric, but the proxy is not overly affected by the broader product team's efforts.
Consider the criterion above, then use the following framework to develop a proxy metric:
Percentage of (new/existing customers) who do at least (minimum threshold of value) by (X period in time).
I’ll apply the model below.
Netflix’s streaming proxy metric at launch
As an example, the proxy metric to validate Netflix’s streaming launch in 2007 was:
the percentage of new customers who watch at least fifteen minutes of streaming content within their first month with the service.
A few notes on this proxy:
We chose 15 minutes because it was the smallest unit of customer value — the shortest TV episode at the time.
We focused on new customers in their first month because we knew that to become an excellent, worldwide service, we’d have to get great at onboarding new customers. Plus, existing customers are not particularly sensitive to change— they overlook new features and don’t like changes.
We evaluated A/B tests at one month to ensure high-cadence learning— that’s when we made go/no-go decisions about new features in A/B tests.
When we launched streaming, the proxy metric was at 5% and, by the end of our first year, we drove the metric to 20% via various tactics (more content, binge-watching, faster download/playback, more platforms). Over time, it was clear that the proxy metric correlated to retention, and in the long-term, we also proved it was a causal relationship.
The proxy metric for personalization
Let’s get back to the personalization example, which is trickier. How do you demonstrate whether a more personalized experience improves retention? What’s your proxy metric for this hypothesis? Like a lot of our data exploration, the conversation began slowly. What metric could we measure to demonstrate members see value in our personalization? What data was available?
There were various hypotheses about what metrics might help us to answer this question, but here’s the proxy we arrived at:
The percentage of new customers who rate at least 50 movies in their first two months with the service.
Here’s the logic: If members rate movies, they do it because they value the recommendations that Netflix provides — members understand that the more movies they rate, the more Netflix will help them find movies they’ll love. Over time, if we provide better recommendations, we’ll begin to see improvements in retention to prove our hypothesis.
We performed lots of experiments and drove our proxy metric from 2% in the early days to nearly 30% five years later. There were a variety of other proxy metrics for personalization — we didn’t rely on just one. For instance, we evaluated how well we did at predicting member tastes through RMSE (Root Mean Square Error), comparing our predicted star ratings with members’ actual ratings. Still, it was improvement against the rating metric that convinced us to keep “doubling down” on our investment in personalization.
Proof that personalization improves retention
By 2012 Netflix demonstrated that personalization did improve retention through a significant, highly controversial A/B test. The team tested a non-personalized version of the site against the highly personalized default experience. The controversy? The engineering team thought it wasn’t necessary to create a purposely poor experience for customers as they felt it was already well-understood that personalization improved retention.
Proxy metrics evolve
Over time, your proxy metrics need to change as the product evolves. For instance, explicit taste data — expressed today by thumbs up/down on Netflix — is much less critical as Netflix relies more on implicit data to predict member tastes. Suppose you watch a new movie for three minutes and quit. This provides a solid signal to Netflix about your movie taste. The proxy metric is no longer the “percentage of new members who rate at least 50 movies in a month.” The personalization metric likely focuses more on the team’s ability to predict your taste— whether you watch a movie they present to you or not. As an outsider, it’s hard for me to guess what the proxy metric is today, but the point is don’t “set and forget” your proxy metrics. You need to reevaluate them over time.
Conclusion
At Netflix, we made decisions quickly, but isolating a viable proxy metric sometimes took 3-6 months. It took time to capture the data, discover if we could move the metric, and see if there was a correlation, then later causation between the proxy and retention. Given a trade-off of speed and finding the right metric, we focused on the latter. It’s costly to have a team focused on the wrong proxy metric.
Eventually, each product manager on my team could measure their performance through one or two proxy metrics we hoped would contribute to retention improvements. The effect was profound: every product leader could describe their job through their metric. They knew when they were doing well/poorly, and the proxy metrics made our debates and decisions much more objective— and much less political. The product leaders evaluated their ability to move their proxy metric through a high-cadence series of A/B tests, enabling them to learn fast.
Over time, each product leader, whether focused on personalization, streaming, customer service— or a half-dozen other “pods” working in parallel—quickly became the smartest person in the room based on their maniacal focus advancing a specific proxy. This is how Netflix managed to improve its high-level engagement metric— through a collection of proxy metrics that eventually moved up and to the right to collectively improve retention.
One last thing!
I hope you found this essay helpful. Before you go, I’d love it if you’d complete my Four Ss below. Bonus points if you do all four. I have listed them in priority order for you.
1) Subscribe! If you’re not a part of the “Ask Gib” community, it’s not too late. Click the button below and you’ll never miss an essay:
2) Share this essay with others! By doing this, we’ll collect more questions and upvotes to ensure more relevant essays:
3) Star this essay! Click the Heart icon near the top or bottom of this essay. (Yes, I know it’s a stretch to say “star” and not “heart,” but I needed an “S” word.)
4) Survey it! It only takes one minute to complete my Net Promoter Score survey, and your feedback helps me to make each essay better:
Many thanks,
Gib
PS. Got a question? Ask and upvote questions here:
PPS. Once I answer a question, I archive it. I have responded to 47 questions so far!
Love the idea of revisiting proxy metrics and not looking at them as something set in stone. :)
You mentioned evaluating performance of a product manager via proxy metric he/she is driving, wondering whether it could lead to a case where PM becomes over-investment in the metric and look for confirmation in data? If so, how to prevent it?
Excellent work Gib!